Applying Statistics to Understanding Petroleum Systems
September 9, 2020 | 5 Minutes Read
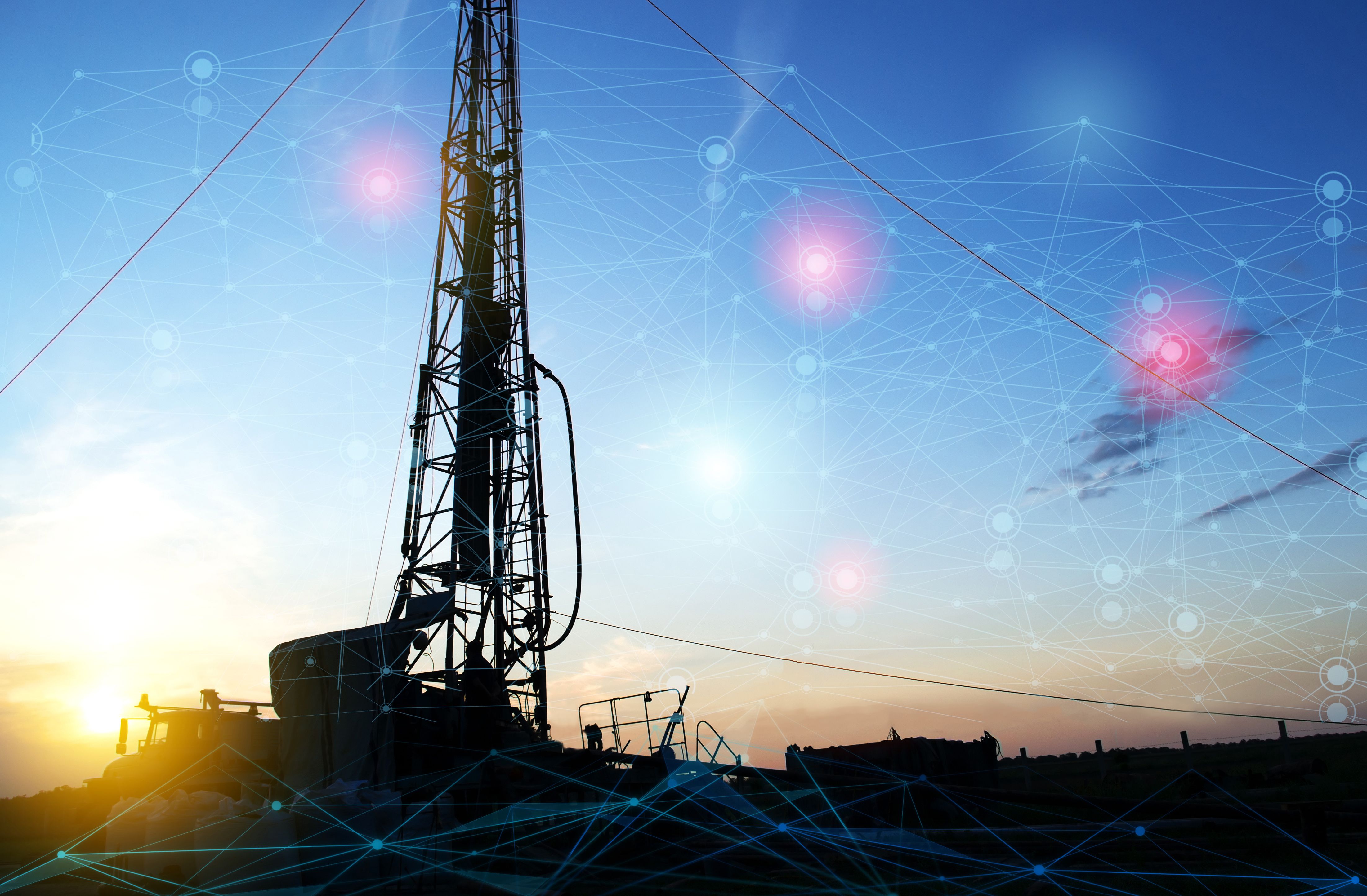
Introduction
There has been a significant increase in interest across the oil & gas industry in ‘Machine Learning’ and ‘Big Data’ over the last several years. The idea being that large datasets can be used to maximize production from resource plays with a prescribed algorithm is attractive, but at the same time, they can be unwieldy and misunderstood without some considerable effort in the machine learning component. While much of the petroleum industry considers this effort to be novel, it is worth reminding practitioners that multivariate statistics, the driving force behind machine learning, have been used across the industry for several decades.
At GeoMark Research our geochemists primarily use 4 statistical algorithms (Pirouette®) to help understand petroleum systems: Hierarchical Cluster Analysis (HCA), Principal Component Analysis (PCA), Soft (Independent) Modelling of Class Analogy (SIMCA) and Alternating Least Squares (ALS). HCA, PCA and SIMCA are all used in the construction of GeoMark’s regional oil studies and oil family definition, from which we determine the identity and character of unknown operator samples. ALS is used to understand mixing contributions and while it is most commonly utilized in ‘production allocation’ studies it is applicable to mixing in general. The following pages outline the basics, with examples, of how we put these algorithms to work.